This is an abridged version of a paper presented at the Fischer Black Memorial Conference on Corporate Risk
Management, UCLA Anderson School of Management, March 29-30, 1996. Figures
appear at the end of the article.
A Framework for Risk Management
in Diversified Financial Companies
by
David P. Greene, Ph.D.
General Electric Company
and
Ross M. Miller, Ph.D.
NatWest Markets
February 1996
I. Introduction
The economic literature on risk management proceeds from the assumption that a formal
valuation model of the assets in which the firm has a position exists and that the risk
exposure of the firm can be computed by determining the sensitivity of the aggregate
assets to changes in a set of parameters that affect the valuation. The Value-at-Risk
approach to risk management has its roots in this approach. Capital-rich, diversified
financial firms, however, have the ability to create new products and markets in the
absence of formal economic models to guide valuation or to calculate aggregate risk
exposure. Such firms by virtue of their size have the unique capacity to bear the
considerable model risk that is often generated by untested products that are
"value-priced" using very informal models with large "tolerance
bands." Once a product is a success in the marketplace; however, the inevitable entry
of imitators can reduce profitability to a point where a formal model is required to avoid
"cream-skimming" by more knowledgeable competitors. The challenge then is to be
able to "shift gears" at the right time, bypassing formal models during the
introductory phase of a product and developing them as the product matures. Furthermore,
because of the diversified nature of the company, the risk created as the product matures
may be related in a variety of ways to risk exposures and prior experiences located
elsewhere in the company.
This paper develops a high-level risk management framework for evaluating the risk
modeling and evaluation process in a financial enterprise that markets several
heterogeneous products lines. Given the current impracticality of a uniform metric for
risk in such an environment, the focus of this framework is the process by which risk (and
its relationship to return) is modeled. It is necessary that this process be on line from
the time of a product's inception if an appropriate measure of risk is to be applied to
the product when it matures.
A key design feature of this framework is to create a sensitivity to the numerous,
sometimes extraordinarily complex, options that are embedded within ordinary financial
products in the minds of key decision-makers. This framework also promotes the development
of an early-warning system to detect problems associated with embedded options and other
shortcomings of informally developed models in order to prevent or contain losses as the
market evolves.
This paper begins with an overview of the framework, examines the five elements of the
framework including examples of their application, and concludes with some comments about
the framework.
II. Overview of the Framework
A guide to the risk management framework is given in Figure 1. The five elements are
numbered to reflect their relative importance. In practice, this framework has captured
all of the salient risk-related features for a variety of financial services businesses
that included insurance, leasing, consumer lending, and commercial lending. Furthermore,
because this approach considers how businesses develop and refine their models as well as
the models themselves, it can evaluate how a business adapts to the risks inherent in its
ever-changing environment.
The first element, embedded options, concerns the options implicitly embedded in
a financial product. These options are important because the failure to recognize them is
a common cause of unanticipated variances in earnings. The second element, feedback,
concerns the formal mechanism for assessing the outcomes of a businesss actions or
customer products-how it shapes and refines its models especially when they go
"off target." The third element, structural dynamics considers the
perspective used by the business to identify and evaluate its marketplace--how the
business frames its models relative to its competitors and the rest of the enterprise. In
Figure 1, structural dynamics is drawn on the fringe of the flow diagram to represent its
meta-level role. The fourth element, model depth evaluates the detail and accuracy
of the business's models. The fifth and final element, model consistency, concerns
the degree to which the models used share a common framework, assumptions or "world
view".
This framework can be conveniently summarized in the form of a one-page business
"report card." The top half of the scorecard summarizes the five elements of the
framework, with the structural dynamics included in the overview. The bottom half of the
scorecard gives three or four major recommendations generated from the examination of the
business within this framework. Detailed supporting material can also be used to back up
the report card.
III. Embedded Options
A fundamental premise of financial economics, that financial commitments can be
decomposed into an underlying set of options, is a completely alien notion to the vast
majority of practitioners in the financial services industry, with the possible exception
of investment-related arenas such as mortgages and annuities. (The capital budgeting view
of embedded options, despite its appearance in business publications, e.g., Sharp, 1991,
has also had limited influence on practice.) The single most important element in
developing an enterprise-level risk management capability is to promote the awareness of
this financial fact of life and to promote its incorporation into both the models and
mindset of the firm. This section will focus on two specific instances where the
option-based view provides critical insights into how risk should be modeled and priced.
The first example of an embedded option is implicit default option that is contained in
all consumer debt and is most important in unsecured debt. This option gives the borrower
statutory means, such as bankruptcy, to limit recourse by the lender. The traditional
approach to modeling default at the individual (and small firm) level is to view these
events as being statistically related to observable attributes of the borrower and the
economic condition of his locale (avoiding discrimination and red-lining either explicitly
or implicitly). Such "scoring" models can be implemented in a variety of ways
using techniques that range from classical statistical inference to neural networks.
Within an options framework, however, default is the result of a conscious (and rational)
decision by the borrower that is made in light of all associated costs and benefits so
that the value of the default option depends on the projected volatility of these costs
and benefits.
Although the rigorous incorporation of optionality into consumer lending is easier to
preach than to practice, a true understanding of the risk of consumer lending is
impossible without taking into account all the options that are given to the consumer in
the context of the lending relationship. Defaults by consumers are not just random
occurrences with observable statistical properties, they are consumer choices. (Fraud,
which lenders tend not to view as a random occurrence, is a limiting case of default by
choice where the borrower disguises his identity in an attempt to shield himself from the
cost of default.) It is of more than passing interest that the increasing use of neural
networks to enhance consumer credit scoring models, may be tightening the indirect link
between the traditional observable attributes used to estimate default and the
option-based view of default. (Cf. Hutchinson, Lo, and Poggio, 1994).
The second example that relates to consumer lending is the early termination option
embedded in almost every loan product, which allows the borrower to "put" the
loan back to the lender at a cost that varies anywhere from a slightly negative cost, when
perverse incentives exist to roll the loan over, to a prohibitively high positive cost,
when the profitability of the loan is linked to the (depressed) market value of the
underlying collateral. The difficulty with these options is that they can be entirely
invisible to the lender. Indeed, such options in the environment where the loan was
initiated may have near-zero value because as options they were then very much
out-of-the-money. The problem is that changes in borrower attributes, unbeknownst to the
lender, can turn such options into in-the-money options. (The process by which such change
can be observed at the enterprise level is covered in the discussion of structural
dynamics later in the paper.)
The sudden emergence of invisible options like the one for early termination has the
potential for a severely negative impact on the firm. Obvious damage occurs when entire
portfolios of seasoned consumer debt are acquired and the option is not figured into the
acquisition price. More subtle damage occurs, however, when options are embedded in loans
that are already on the books, which financial theory tells us should already be reflected
in the value of the firm. In this case, the sudden, unexpected inflow of cash and booking
of losses associated with early termination can disrupt the financial processes of the
firm, even leading to a downgrading of its debt in cases where the rating agencies require
observable market feedback to value the firm properly.
The problem of hidden embedded options is often exacerbated by the tendency of lenders
to ignore the status of borrowers once a loan is made as long as the payments are received
in a timely manner. Despite the cost savings that can result, the potential for disaster
from failing to reevaluate certain loans periodically becomes more apparent when the
optionality of the loans is acknowledged explicitly.
IV. Feedback
In the previous section we evaluated one aspect of efficiency, that all options
embedded in a product were properly valued. In this section we examine another aspect,
that all the information related to the past performance of a product is used effectively
in the decision-making process. Although most financial services companies collect
enormous amounts of raw data, the decision of what data to collect and how to process it
is often flawed. Once data collection has been organized in a certain way, it tends to
become institutionalized and, therefore, exceptionally resistant to change. Not only can
generating the resulting reports be irrelevant and time consuming, but the sheer excess
desensitizes decision makers to useful signals. For reviewing risk, the issue is whether
the information the business is collecting can be used and actually is being used to help
them better understand and model their market.
Useful feedback lies on three dimensions: timing which considers the duration of
actions, effects and observations, encoding, which considers which data are
captured and how they are reported, and evaluation which looks at the incentives
that influence interpretation of the reported results.
Timing relates to how quickly we know of a customer's choice, such as the exercise of
an option, after it occurs. Timing is often effected by how readily the business's
information systems can distinguish different states of nature and compile them in a
usable form. Timely and accurate feedback can be especially difficult from the corporate
perspective if a variety of disparate businesses have been acquired over time, because
different "legacy" systems can be based on incompatible partitions of the states
of nature.
More problematic for feedback is the duration of an event. As was pointed out in the
previous section on embedded options, the duration of payment or benefit terms in a
financial product such as mortgages or leases can allow for changes in the underling
preferences and alternatives available to the customer. If those underlying conditions are
not considered or monitored, the negative consequences can be rapid and severe. While due
diligence and seasoning are standard practice in some markets, they are often overlooked
by many businesses or products which have fundamentally similar structure. For new
products or markets, the desire for rapid growth can create potentially serious exposure
which goes unrecognized.
A practical dilemma is the contradiction of growth and consistency--shareholders want
to see perpetual, sometimes unrealistic growth, but they want it to be stable. In
practice, growth tends to be a volatile mix of success and failures. Using entirely
acceptable accounting procedures, such as the timing and booking of sales or the
allocation of costs, the business can report smooth results. Unfortunately, those same
reports are often used to evaluate the marketplace and do not clearly reflect the
underlying conditions needed to assess cause and effect (Bushman and Indjejikian, 1993).
Also, without the addition of contextual information, such as prevailing economic
conditions at the inception of a market action relative to current economic conditions, a
business would lack the necessary feedback to refine its models, even though from an
accounting standpoint it would appear to be profitable.
This raises the difficulty in how feedback generates guidance and reinforcement. Even
if systems are set up to provide useful feedback about risk, unless the incentive
structure rewards an appropriate risk-adjusted measure of return, the feedback will have
no effect on the process. Indeed, with insufficiently sophisticated systems, it may be
impossible to generate an incentive-compatible measure of return.
Compounding the problem is the perception that if things are going well financially
relative to an arbitrary benchmark, what we are doing is all right; hence, we know what we
are doing and should continue doing it. As we noted earlier, with new products or markets
large firms bear the risk of informal models by employing large tolerances. Over time,
with the appropriate use of feedback, the models should become more accurate and the
tolerances more precise. In practice, there can be a tendency not to refine models after a
successful production introduction because the initial success can be misperceived as
model accuracy which reinforces the initial informal model (Einhorn and Hogarth, 1978).
For example, the idea of being conservative about risk by underwriting to target zero
loss levels, which is unexplored in the academic literature but occurs in practice, can
result in setting thresholds too high. The business may be successful at screening out bad
credit risks initially but is leaving opportunities for competitors to get a toehold in
the market. By not developing an accurate model of the market, the business can end up
"conservatively" defending a share of a diminishing market when, in fact, the
market is not diminishing but has simply transitioned into a different form.
V. Structural Dynamics
A third dimension in assessing enterprise-level risk, structural dynamics, requires
understanding the context and environment in which the models are evaluated, both from the
perspective of the parent company as well as the individual business. Most businesses will
categorize themselves by their industry, sub-industry, product, customers and competitors.
In some cases those designations are critical for regulatory purposes and constrain the
firm's behavior; however, in most cases the categories are arbitrary, overlapping and
self-selected. While such classifications are useful for providing comparisons or referent
standards they can led to distortion if they provide too narrow a perspective for
modeling.
Problems arise when a business imputes some absolute association to its self-perception
and its products; for example, it views itself as a bank because it has "bank"
in its name and offers banking products. As long as the market accepts those definitions
the perspective is appropriate. But if customers start viewing the business merely as the
utility it provides (e.g. interest and liquidity) a perceptual shift can occur as new
information and alternatives become available. Perceptual shifts can be especially acute
if legal or regulatory barriers exist in one market but not the other, as was the case
when Merrill Lynchs original Cash Management Account was able to sidestep banking
regulations to offer better value for similar services (Farkas, 1991). Conversely, some
businesses face the risk of vanishing overnight though the passage of a single piece of
legislation, e.g., The Tax Reform Act of 1986.
A major competitive advantage that diversified financial services firms possess, at
least in theory, over more specialized firms is that aggregate shifts in consumer behavior
detected in one business can serve as an early warning system for related businesses. For
example, the structural shifts that led to unprecedented mortgage refinancing in the late
1980s and early 1990s were a harbinger of things to come in other consumer financial
businesses with embedded early termination options.
VI. Model Depth
Implicit in all models is the partitioning of the possible states of nature. Model depth
is the fineness and appropriateness of this partition. Like feedback, the depth available
to a model can be highly dependent on the information systems that provide the data for
the model.
Financial service firms apply a wide range of models to estimate credit risk, residual
value (for leased assets), and profitability (for credit cards). From a statistical
standpoint, assessing depth is relatively straightforward where clear objectives can be
specified for quantitative models, unfortunately this is rarely the case. As noted in the
previous section, the choice of what to model and how to model it is usually a reflection
of standard practice in the industry. Although such practices are perceived as
conservative since they represent the prevailing standard, they can reinforce a restricted
perspective making it harder to detect structural shifts and to differentiate the
businesses product.
While a top-down perspective of the business should motivate model construction with
bottom-up validation through empirical data, in practice, the models usually evolve in a
piecemeal fashion to address specific concerns or requirements. In many cases the
inclusion of certain features are historical artifacts which may no longer be appropriate
but which are institutionalized in the systems and accounting.
Consider the problem of new products that are developed as extensions of an existing
product to satisfy additional needs of existing customer. Often, the business will apply
existing models without fully considering if the underlying behavior of the customer or
market will be the same. As noted previously, even if feedback is provided, it can
reinforce misperceptions leading to false confidence and potential pitfalls of embedded
options among other risks.
VII. Consistency
The final element examined in assessing enterprise-level risk was the consistency of
the quantitative models employed in the decision-making and accounting processes. The
level of consistency that is implicit in the theoretical financial literature is
impossible to achieve in a diversified financial enterprise. The decentralization of
decision-making required for the enterprise to function guarantees inconsistency in the
models and their underlying assumptions in both their construction and implementation. It
is financial folklore, for example, that even Wall Street's most prestigious firms with
unlimited access to state-of-the-art modeling techniques can have a dozen or more
different, mutually-inconsistent models of the term structure of interest rates in
operation simultaneously.
Although such inconsistency can have a rational basis; in reality, it serves as
evidence of potentially serious problems when the inconsistencies cannot be rationalized.
The gratuitous failure to create a consistent framework for the models within a business
is strongly symptomatic of problems within the models themselves beyond those directly
attributable to the inconsistency. Although Ralph Waldo Emerson is well-known for saying
that "a foolish consistency is the hobgoblin of little minds," in this case
consistency is not at all foolish but rather a superb signal of overall model quality.
Before concluding, there is an interesting rationalization of model inconsistency that
needs to be acknowledged because it reflects an interesting divergence between the worlds
of theory and practice. In more than one instance the authors have encountered
practitioners who claim to consciously use inconsistent models as a means of risk
diversification, so that if one model turns out be flawed, the entire holdings of the
business are not affected. The suggestion that such diversification can also be
intentionally achieved within a consistent meta-model is not well-received. In part, this
poor reception appears to be related to a subtle cognitive bias that is very difficult to
remedy; however, it is also related to practical difficulties in achieving consistency
across models with fundamental different functionality. For example, calibrating models
that project regional business conditions for different types of consumer loans made
within a single business so that they match on a region-by-region basis can be an
impossible task when the models rely on underlying states of nature that are orthogonal
across models.
VIII. Conclusion
The risk management framework described in this paper has proved in practice to be an
excellent mechanism for understanding how risk manifests itself within individual
businesses and how risks are related throughout the enterprise. In many instances adoption
of this framework has led to immediate and substantial changes in the risk management
modeling procedures within a business. Not surprisingly, the notion that "everything
is an option," which is second nature to financial economists, is difficult to
incorporate into the mindset of many practitioners; however, this framework has made some
progress in that area. The subtle ways in which optionality can manifest itself in
financial products requires a deeper education than that which can be provided by this
framework.
References
Bushman, Robert M. and Raffi J. Indjejikian, "Stewardship value of 'distorted'
accounting disclosures," Accounting Review, Vol. 68, No. 4, 765-782, October
1993.
Einhorn, H. and Hogarth, R., "Confidence in judgment: persistence of the illusion
of validity," Psychological Review, Vol. 35, No. 5, pp. 395-416, 1978.
Farkas, Mitchell S., "The account that transformed a brokerage into a bank," Financial
& Accounting Systems, Vol. 6, No. 4, pp. 8-12, Winter 1991.
Hutchinson, James M., Andrew W. Lo, and Tomaso Poggio, "A nonparametric approach
to pricing and hedging derivative securities via learning networks," Journal of
Finance, Vol. 49, No. 3, pp. 852-889, July 1994.
Sharp, David J., "Uncovering the hidden value in high-risk investments," Sloan
Management Review, Vol. 32, No. 4, pp. 69-74, Summer 1991.
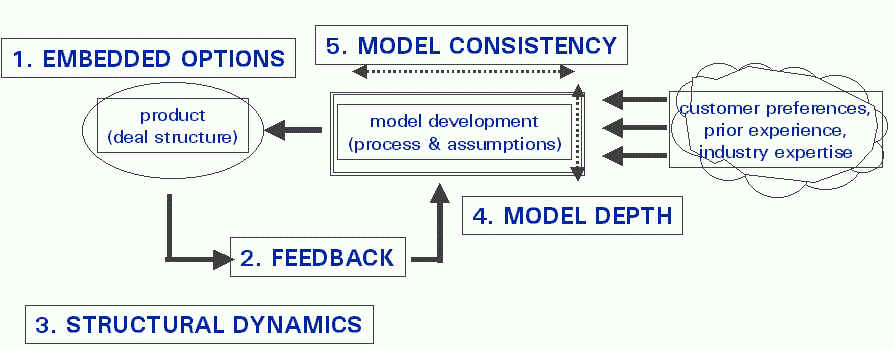
Figure 1: Guide to the Framework
Copyright © 1996 by General Electric Company and
Miller Risk Advisors.
All rights reserved.
|